Predictive Analytics for Port Optimization
By Awake AI
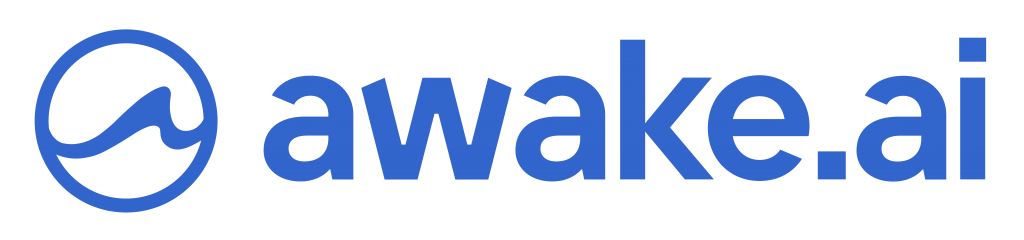
The iNGENIOUS project considers next generation IoT solutions for the universal supply chain, where focus is especially around maritime logistics (sea and hinterland transports and port operations). There, sea ports are important nodal points, whose operational efficiency has a significant effect on the surrounding multimodal logistics.
The table below presents examples of IoT, edge processing, and analytics applications in maritime logistics around port operations. Use cases in iNGENIOUS (link) cover many of these, from remote control of AGVs via tactile IoT to resource tracking in sea and hinterland transport to predictive analytics for asset management and operational planning. In this article, we focus on use of predictive analytics in ports and surrounding multimodal logistics for improved schedule estimation and related planning.
Sea | Port | Hinterland | |
Autonomous & remote controlled vehicles | MASS (Maritime Autonomous Surface Ships) | AGV (Automated Guided Vehicles) | Autonomous trucks |
Monitoring, situational awareness | Vessel and container tracking, smart fairways | Cargo and resource tracking and monitoring, digital twins | Automatic gate monitoring systems, vehicle tracking |
Predictive asset management | Predictive engine health and energy management | Predictive machinery health management | Predictive engine health and energy management |
Scheduling, optimization | ETA (Estimated Time of Arrival) predictions, JIT (Just In Time) arrivals | Cargo operations prediction, RTA (Requested Time of Arrival) | ETA, JIT |
What do we mean by predictive analytics in this setting, and why is it needed? Generally, predictive analytics means using past and current data with statistical analyses, computational models, and algorithms, to produce predictions about future events. Relevant targets for predictive analytics in maritime logistics include times and durations of various events and processes (such as vessel arrival times or cargo operations durations), resource requirements (e.g. bunkering, tug assistance), and future courses of action (for example destinations and future routes of vessels). These are all necessary information for planning operations efficiently. However, it is still common that such data are not reliably available or sufficiently shared between actors in the logistics chain who would benefit from the information. For example, typical data quality problems regarding vessel scheduling are outlined in this this blog-post.
Planning operations efficiently requires situational awareness, or information on the current state of affairs, capability for predicting how current processes will carry out, and adapting plans according to foreseen changes. A common example in maritime logistics is port call planning, where allocation of resources such as pilotage, fairway usage, tugs. berth places, cargo handling equipment, storage facilities, and personnel need to be continuously monitored and planned according to related vehicle and cargo flows. For many ports, this is still a manual process where planners gather related information from multiple sources providing data of variable accuracy and timeliness. This becomes challenging especially in the presence of congestion and unexpected changes in schedules and resource availability. Modern analytics solutions can help optimize the planning process by integrating all relevant information to the same environment, improving data quality and predictions, and providing alerts and possible optimization suggestions for the planners.
The figure above illustrates common applications for predictive analytics and other machine learning related to port call planning and monitoring port operations. To provide support for planning, it is beneficial to continuously monitor relevant vessel (or hinterland carrier) traffic to predict which vehicles are currently on their way to the port, which routes they will take, and when they will arrive. Furthermore, to enable estimating resource availability at the port, durations of cargo operations can be predicted both before vessel arrival to port and with real-time progress information taken into account once the operations have started. As outlined above, such predictions involve many intermediate computations and component models, and require integrations to multiple information systems, from public sources to commercial data providers to port operators’ proprietary systems.
As practical examples of predictive analytics regarding vessel scheduling, the figures below illustrate predictions performed in the Awake.AI smart port platform. The upper figure shows a snapshot of incoming vessel traffic for the port of Rotterdam identified on June 3, 2021 by classifying destination reports provided by vessels globally through the Automatic Identification System (AIS). The lower figure focuses on a single vessel from this group, illustrating the predicted route the vessel will take to Rotterdam, along with the Estimated Time of Arrival (ETA). In this figure, the ETA shown in white is provided by the vessel, whereas the time in blue is computed by Awake.AI based on the vessel’s current situation and our prediction models. The specifics of our ETA prediction approach are discussed in this blog-post.
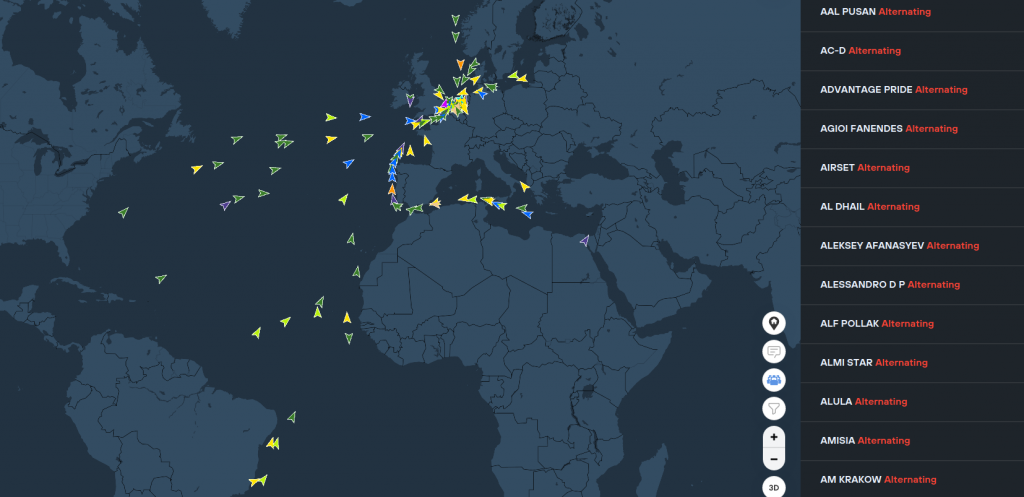
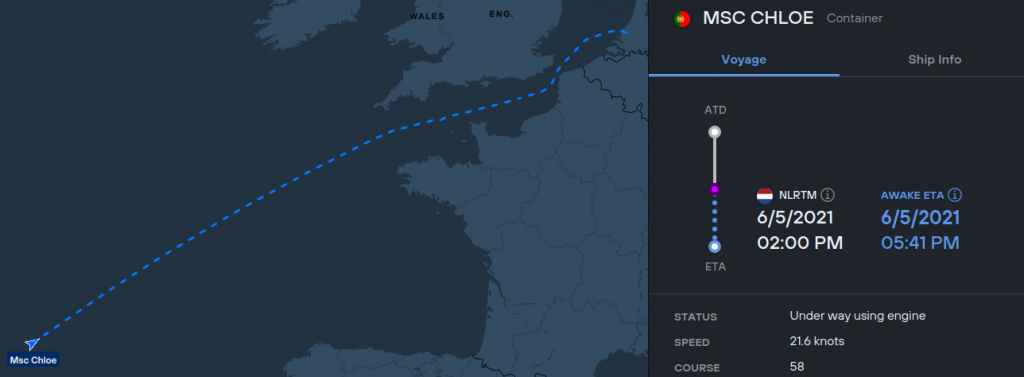
Vessel arrival time predictions are typically used as part of planning other operations or schedules, such as cargo discharge and loading, hinterland pickups, or even changes to the vessel arrival schedules themselves e.g. in order to optimize fuel consumption. For holistic optimization of port operations, it is beneficial to combine ETA predictions with predictive models for related processes such as cargo operations.
The figure below illustrates how combining ETA and cargo operations predictions can be used to facilitate Just in Time (JIT) arrivals, meaning that ships arrive to port just before they can be accommodated, instead of rushing to wait at anchor and consuming additional fuel in the process. This is seen by the International Maritime Organization (IMO) as an important objective in reducing emissions from shipping (link). In the example below, we have predicted for one week ahead the arrival schedules of vessels to a given berth (distance from berth shown in the vertical axis, time in the horizontal axis) and the durations of operations at berth for those vessels (horizontal segments at zero distance). Predicting such schedules in advance enables adjusting vessel speeds towards just in time arrival, if a suitable communication channel is available for providing Requested Time of Arrival (RTA) messages for the vessels.
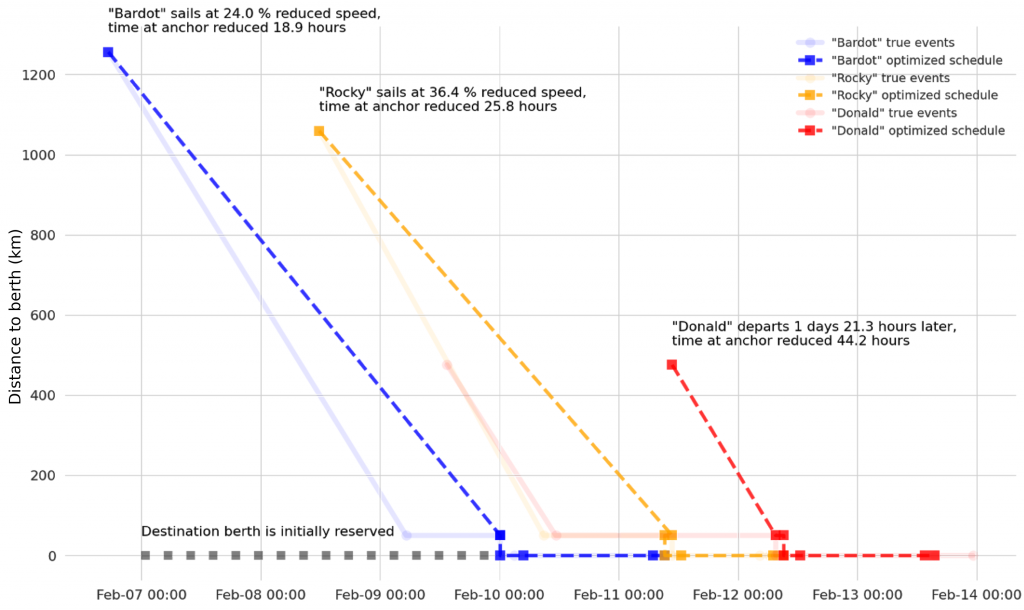
Currently, there are still many factors which may prevent or complicate implementation of JIT arrivals, as considered in the IMO document linked above. In ports suffering from congestion, i.e. inability to process vehicles or cargo at the necessary rate, this can lead to significant anchorage times for vessels waiting to enter the port. The figure below illustrates anchorage times in a busy port, showing an increase in the level of congestion (the bars in this Gantt-type chart represent waiting times for individual vessels, separated by the berths they are entering after anchorage). If the ship schedules cannot be influenced, then predictive analytics and related optimization can be applied to allocate available resources with the objective of minimizing the total costs from unavoidable waiting times.
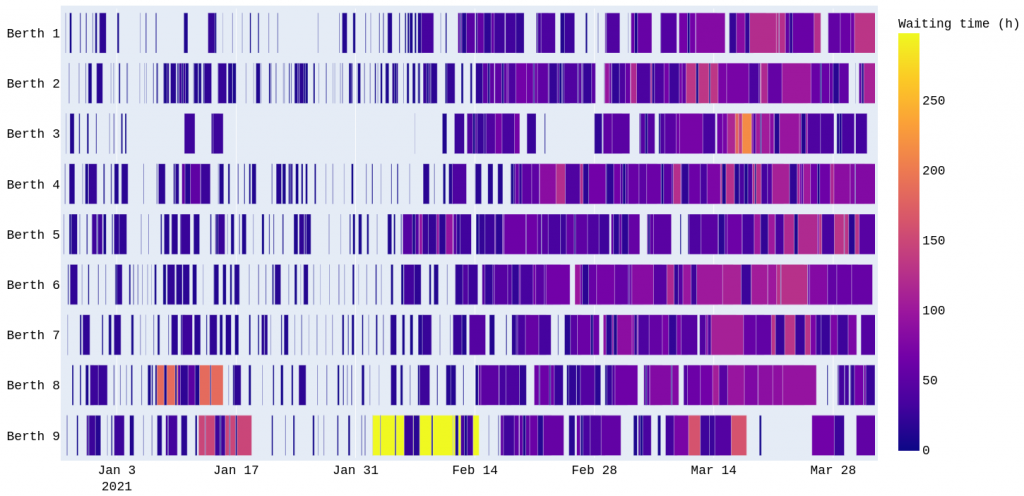
In the iNGENIOUS use case “Situational understanding and predictive models in smart logistics” the objective is to apply predictive analytics for modeling the relationships between cargo flow rates related to vessel port calls, which can be continuously monitored and predicted as considered above, and truck congestion in the port area. Being able to predict truck congestion and related increase in truck turnaround times is valuable for scheduling hinterland transports and taking practical steps to reduce landside congestion. We will return to this use case in more detail in a later entry of this blog.